Ani Sridhar
Postdoc @ MIT Mathematics
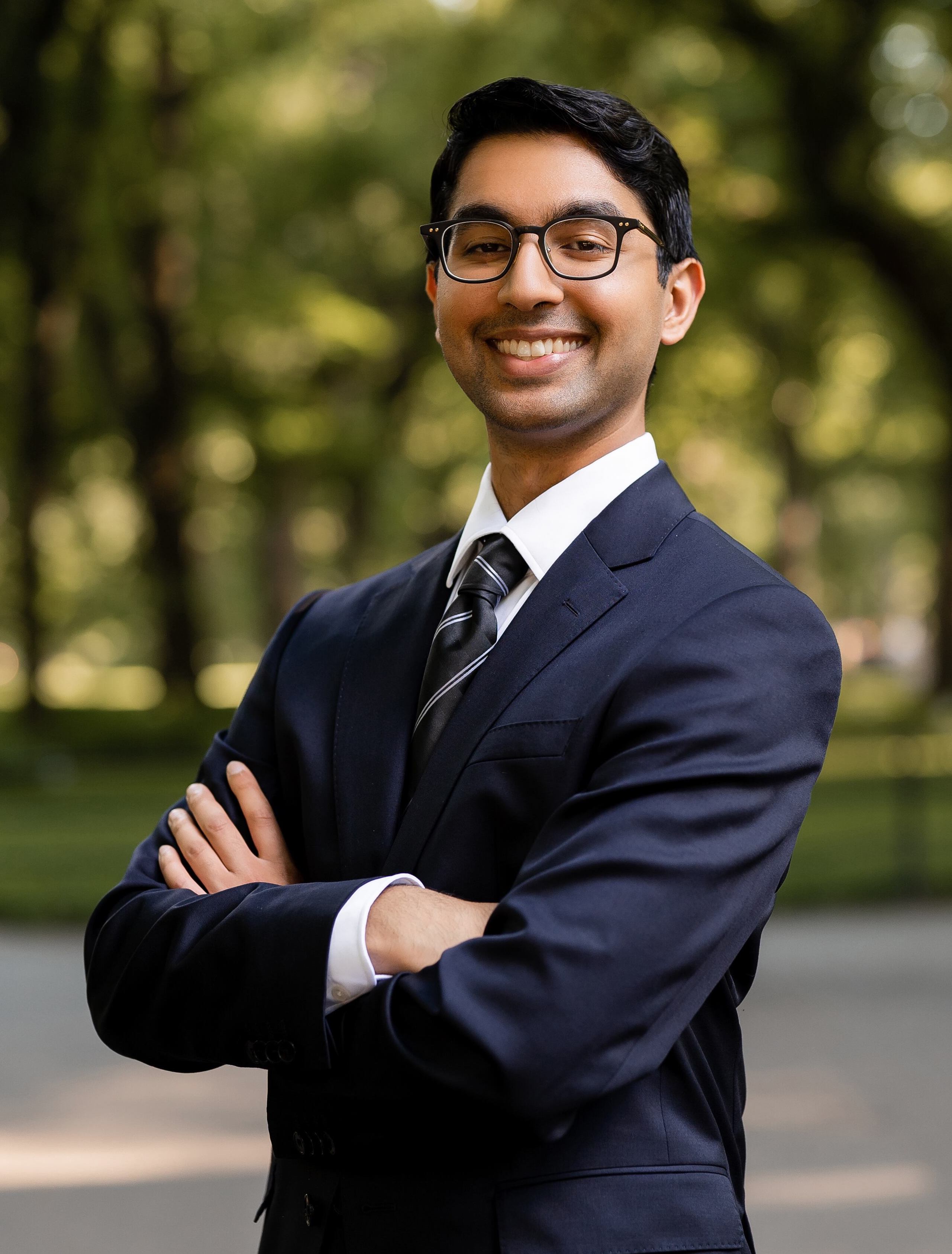
MIT 2-246A
anisri@mit.edu
I am a postdoctoral associate at the Mathematics Department at MIT, working with Elchanan Mossel. Previously, I completed my PhD at Princeton’s Department of Electrical and Computer Engineering, where I was advised by Miklós Z. Rácz and Vince Poor. My research uses tools from probability, statistics and graph theory to tackle fundamental challenges in network analysis, causal inference and epidemiology. Recent and ongoing research directions include:
- Graph matching. How can structural similarities be found across networks, and what can we learn from the commonalities? My research characterizes the precise information-theoretic thresholds for the possibility or impossibility of this question and related ones, such as community detection or clustering.
- Modeling and mitigation of network cascades. How does a cascading process – such as misinformation in social networks, malware in computer networks, or a virus in a human population – evolve over time? And how can we learn about the ongoing spread before such cascades cause too much damage to society at large? My research develops the underlying theory behind new aspects of cascade models (e.g., viral mutations, mask-wearing) and designs data-driven statistical methods for quickly learning key information about cascades.
For additional details, you can check out my CV or my Google Scholar page.
news
Jun 30, 2024 | New paper on finding super-spreaders in network cascades to be presented at COLT 2024. Joint work with Elchanan Mossel (MIT). |
---|---|
Mar 14, 2024 | Speaking about quickly tracking network cascades at a session on Advances in Sequential Analysis and Change Point Detection at the CISS 2024 conference. |
Nov 16, 2023 | Paper on mean-field approximations for stochastic processes on networks published in the SIAM Journal on Control and Optimization (SICON). Joint work with Soummya Kar. |
Oct 17, 2023 | I will be giving a talk and chairing a session at the Informs 2023 conference on Community Detection in Networks. |
Sep 19, 2023 | New paper on the average-case and smoothed complexity of graph isomorphism. Joint work with Miki Rácz and Julia Gaudio. |